CUSTOMER PREDICTIVE ANALYSIS – CPA
Let computation power select your consumer mailing list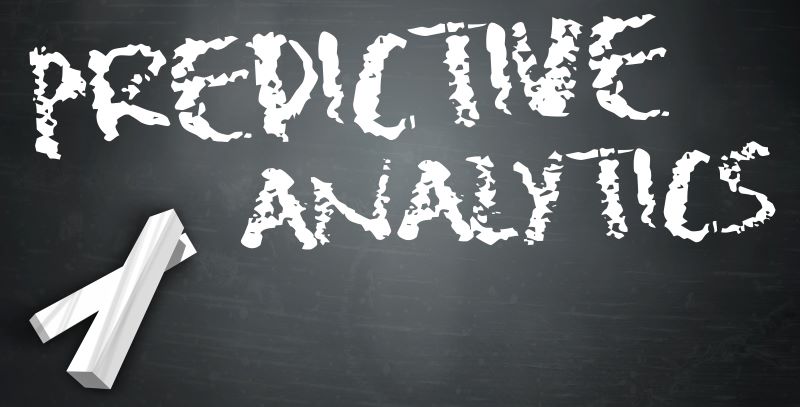
It’s time to delegate the delicate task of selecting your consumer mailing lists to a computer. Study after study is showing that computation power is better than intuition and experiential expertise. Moneyball showed how computation power can select baseball talent better than professional scouts. Grandmasters can no longer beat a computational chessboard. Trial lawyers are using computation power to select juries. The examples are endless. Bottom-line, it’s time to let computation power select your consumer leads. To help delegate this task, we offer the CPA … Customer Predictive Analysis.
In a nutshell, the Customer Predictive Analysis (CPA) is a statistical model that compares your customer file to a prospect universe. By crunching endless iterations and permutations – computation power will find highly qualified prospects that look-like your best customers. After all the crunching the Model will rank prospecting names by propensity to respond based on how closely the prospects resemble your best customers.
The Customer Predictive Analysis (CPA) can:
Optimize your response rates
Reach more qualified leads
Reduce mailing cost by allowing prioritizing of leads
Steps in building your CPA:
1. We begin by matching your customer file to the National Master Consumer Database. When a match is found we temporarily append an extensive array of demographic, psychographic, behavioral, and transactional data to the customer record.
2. Next we select a random sample of non-customers from the National Consumer Database to compare with your customers. We then randomly split your customer file into a Model-Building Sample file and a Validation Sample file. Then we use chi-squared test of significance to isolate the most significant variables that differentiate your customers from non-customers in the National Consumer Database. Using stepwise linear regression, we further refine the list of significant characteristics and remove highly correlated characteristics.
3. Using logistic regression, we then assign weights to each of the most significant characteristics. Then we calculate an overall score for each non-customer from the Master Consumer Database by adding up all the regression weights for every significant characteristic for each individual. *Pre-select criteria may be applied from this point on.
4. We then sort and divide the non-customers into ranks based on their overall scores. Your customers are then scored and ranked using the score cut-offs for each rank. This score measures how similar the Prospect Names are to your customers.
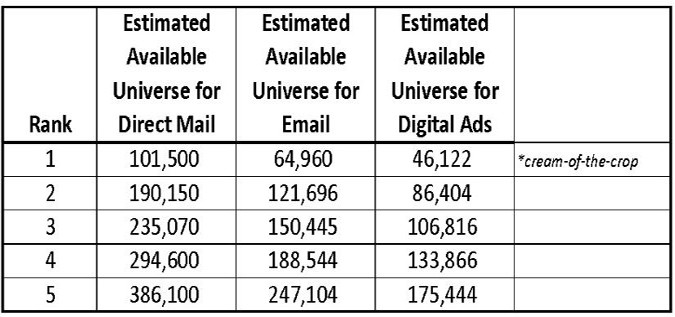
Other links related to consumer list profiling.
LEAD SCORING - Click Here
CUSTOMER PROFILE ANALYSIS REPORT - Click Here
LIFESTYLE DATA APPEND - Click Here
CONSUMER DATA APPEND - Click Here